This is a post about hot hands in basketball. But first, some relevant history:
The single most controversial topic ever broached here on The Big Questions was not Obamacare, or tax policy, or the advantages of genocide, or the policy treatment of psychic harms. It was this:
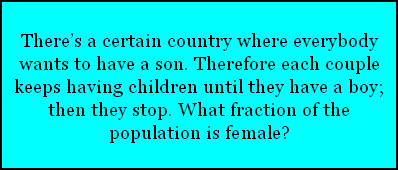
The answer, of course, is that you can’t know for sure, because (for example) by some extraordinary coincidence, the last 100,000 families in a row might have gotten boys on the first try. But in expectation, what fraction of the population is female? In other words, if there were many such countries, what fraction would you expect to observe on average?
The “official” answer — the answer, for example, that Google was apparently looking for when they posed this as an interview question — is that no stopping rule can change the fact that each birth has a 50% chance of being either male or female. Therefore the expected fraction of girls in the population is 50%.
That turns out to be wrong. It’s true that no stopping rule can change the fact that each birth has a 50% chance of being either male or female. From this it does follow that the expected number of girls is equal to the expected number of boys. But it does not follow that the expected fraction of girls in the population is 50%. Instead, that expected fraction depends on the country size, but is always less than 50%.
If you don’t see why, I encourage you to browse the archive of relevant blog posts. If you still don’t get it, I encourage you to keep browsing. Whatever your objections might be, you’ll find them addressed somewhere in the archive. I’m not interested in relitigating this. I will, however, happily renew my offer to take $5000 bets on the matter, on the terms described here. Last time around, all takers changed their minds before putting any money on the table.
Now let’s get to the hot hands.
Thomas Gilovich, Robert Vallone and Amos Tversky (I’ll call them G, V and T) made quite a splash back in 1985, with a claimed debunking of the hot hand “myth”. According to the authors, a player who has just made his foul shot is thereby rendered neither more nor less likely to make the next one.
Now a new paper by Joshua Miller and Adam Sanjurjo (call them M and S) claims that G, V and T drew the wrong conclusions because they made exactly the same mistake that leads to the “official” but wrong answer to the boy/girl problem.
I’m particularly delighted by this, because back in 2010, when we were debating the boy/girl problem on this blog, a number of people defended the official answer vigorously, then realized they were wrong, and retreated to a position of “but it doesn’t matter for anything anyhow”. My experience in the classroom tells me that if you want to convince people that an idea does matter, the most effective strategy is to show them an application to sports. Where were M and S when I needed them five years ago?
Anyway—here’s why the two problems are the same problem. One of the several tests in the GVT paper consisted of placing ballplayers a distance from the basket where they could be expected to make just 50% of their shots. (This distance was, of course, different for every player). The players then made several attempts, and the researchers asked whether the observed sequence of hits and misses looked statistically identical to what you’d get from a sequence of fair coin flips. If so, then because we all know there’s no such thing as a hot hand in coin flips, we can conclude that there’s no such thing as a hot hand in basketball (or at least in this particular experimental setup).
So — what does a series of, say four coin flips (corresponding to four foul shots) look like? It looks like one of sixteen things: HHHH, HHHT, etc. If you’re trying to flip heads, a “hot hand” should mean that the sequence HH comes up more often than the sequence HT. If you don’t have a hot hand, then all sixteen sequences should be equally likely, and the frequencies of HH and HT are as follows:
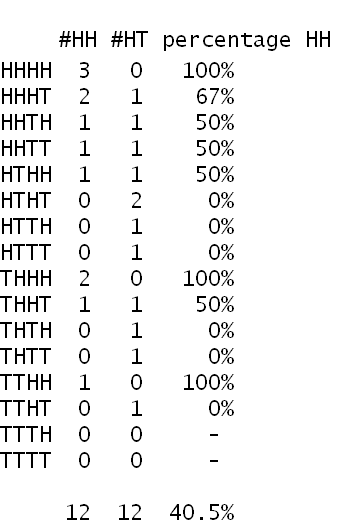
Sure enough, across the sixteen sequences, HH and HT occur equally often — a total of 12 times each. So if you put the players at their foul lines, let each one take four shots, count the HHs and the HTs (where “H” now means “made it” and T now means “missed”), and if there are no hot hands, you should see about the same number of each.
But G,V and T did something a little different. For each player they computed the ratio HH/(HH+HT) — and, because they fell prey to exactly the same mistake that trips up so many would-be solvers of the boy-girl problem, they expected that for the average player, this should come out to about 50%. That’s not right. In fact, as the chart shows, it comes out to about 40.5%.
When G,V and T observed an average success ratio of about 50%, they said “Yup, that’s what we expected all along. No evidence of a hot hand here”. What they should have said was: “Wow! We expected 40.5%, not 50%. That’s a big difference. Only a hot hand could account for this.”
(Caveat: This was only one of the tests that G,V and T performed. I haven’t thought about which of their other tests were or were not affected by this slip up.)
Of course, if The Big Questions had been around in 1985, G, V and T would surely have been avid readers who had worked out the boy/girl puzzle and learned to avoid this mistake. Science marches on.
Shouldn’t #TT be #HT in your table?
Sorry that I have to go back to your boy/girl brainteaser.
But in my understanding, the question asked “What fraction of the population is female” NOT “what is the average fraction of a family that is girl”
So I would say the answer is simply Total number of girls in the whole country / Total number of people in the whole country = 0.5?
So that turns out to be a problem of literal definition instead of a mathematical problem?
actually ignore me. I just figured it out. Sorry.
I think I can just about follow this, under the following assumptions:
1 A “foul shot” is something that’s done by one player by themselves with the game paused, not something that happens in the course of normal play, and which can quickly be determined to have succeeded or not; and “making a foul shot” is succeeding in it. (Sorry; I’m from the UK and haven’t encountered much basketball. “Making a foul shot” initially sounded to me like it meant ‘playing in a way which isn’t allowed by the rules’.)
2 In the table, the column headed ‘#TT’ should be ‘#HT’.
With the country boy/girl case, the exact answer is always different from 50%, but with a decent sized country it is pretty close. The difference between 50% and the actual expectation is small enough to ignore for practical purposes. In this example it is clear that the difference is significant and cannot be ignored.
I seem to remeber an extra half boy in the last lot – can that concept help us here?
There are differences between the earlier problem and this one. With two throws we have HH, HT, TT, TH only. HH occurs once, HT occurs once so each occurs the same. The average HH/(HH+HT) is 50% in this case – we either get 1/1, 0/1 or 0/0. If G,V and T got a series of players to take two shots, then worked out HH.(HH+HT), they would expect 1/2.
For three throws I calculate 41.7%. For four throws it is 40.6. Unlike the last problem, this seems to be getting smaller with more throws. The other one approached 50% with bigger countries.
From a casual obsevation, this seems to be because you cannot excede 100%. The more throws you have, the more ways you can have 100%, so if you have HH:HT of 1:0, 2:0, 3:0 or more, you still only get 100%. So those three HH in the last one only get counted the same as that single HH int he first one.
So what is the limit for an infinite number of throws, and does this have any significance? Or have I mis-understood the whole thing?
The title of the third column should be #HT and not #TT
Thanks to the several of you who pointed out that my second column was mislabeled. Fixed now.
My first thought on the boy girls things was:
50% of families will likely have a boy first birth and stop breeding.
Even if all future births are 50% boy/50% girls this will never counter the 50% of families who have a boy as first-born and stop breeding, so the ratio of girls will always be less than 50%.
This seems way simpler (to me anyway) than the explanation actually given. Is it wrong?
O0pps – obviously its wrong – (must remember to keep my first thoughts to myself in future !)
Harold:
For three throws I calculate 41.7%. For four throws it is 40.6. Unlike the last problem, this seems to be getting smaller with more throws.
I get these numbers:
For 3 throws: 41.7% (same as you)
For 4 throws: 40.5% (as in post)
For 5 throws: 40.8%
For 6 throws: 41.6%
For 7 throws: 42.5%
For 8 throws: 43.3%
For 9 throws: 43.9%
For 10 throws: 44.5%
For 11 throws: 45.0%
For 12 throws: 45.5%
….
I think I’m starting to get my head around this, but I still haven’t fully grokked it. The following sentence is still true, right:
“For any given flip, if all I know about it is that the previous flip was heads, the probability that this flip is heads is 1/2”
Does anyone have a simple statement which describes the event that happens 40% of the time?
John Faben:
For any given flip, if all I know about it is that the previous flip was heads, the probability that this flip is heads is 1/2
This is correct.
Does anyone have a simple statement which describes the event that happens 40% of the time?
If you flip four times, and take the percentage of “heads” flips that are followed by another heads flip, that percentage, on average over many four-flip sequences, is 40%.
I think people get tripped up by thinking of E(X)/E(X+Y) as opposed to E(X/(X+Y)). Once you write them out and are clear about what you’re asking for it’s not hard to understand.
Amusingly the expected surplus of boys to girls E(Y-X) is still zero, even as the ratio stays below 50%.
One way to think about it is:
Suppose you have 2 heads and 2 tails in a sequence. What’s the probability that the first head is followed by another head? It’s not 1/2. It’s 1/3, since, after the first head, what must follow comes randomly from what remains, which is one head and two tails.
If that’s not obvious, imagine picking three-digit lottery numbers. Out of 2000 picks, “222” appears twice, as expected. What’s the probability that “222” follows “222”? Not 1/1000, but 1/1999.
#15: “It’s 1/3, since, after the first head, what must follow comes randomly from what remains, which is one head and two tails.”
If I understand the problem as you set it up, the statement above is only true for the 50% of cases where the first coin in the four coin sequence is heads. However, in the 50% of cases where the first coin is tails, this is not true since now there is at most one tails left to follow the first heads.
Another way to look at it, the “first” heads can be in one of three places: Coin 1 (1/2), Coin 2 (1/3), or Coin 3 (1/6). If it’s Coin 2, there’s a 50/50 chance the next coin is heads (since there is one of each remaining) and if the first heads is Coin 3 there’s 100% chance the next coin is heads for obvious reasons.
So you have (1/2)*(1/3)+(1/3)*(1/2)+(1/6)*1=1/2.
You can also just list the six ways: HHTT, HTHT, HTTH, THHT, THTH, TTHH and see that half of them have the first H followed by another H.
As someone on the original thread suggested, if people are still confused about the boy-girl problem, imagine a simplified population with a single family, which also adheres to the rule that they won’t have more than 2 kids no matter what. (This avoids the problem of summing infinite series.)
If their first child is a girl, then there is (let’s say) an 80% chance that they will try for another child. Then you have:
– a 50% chance their first and only child will be a boy
– a 10% they will have one girl, and then stop
– a 20% chance they will have a girl, and then a boy
– a 20% chance they will have a girl, and then another girl
Now in this case:
1) The *expected* proportion of girls is 0.5*0 + 0.1*1 + 0.2*0.5 + 0.2*1 = 0.4.
2) The expected number of girls is 0.5*0 + 0.1*1 + 0.2*1 + 0.2*2 = 0.7.
3) The expected number of boys is 0.5*1 + 0.1*0 + 0.2*1 + 0.2*0 = 0.7.
So you can have a case where the expected proportion of girls is not 0.5, but the expected number of girls is the same as the expected number of boys.
The intuitive reason why those can both be true at the same time, is that in the one-child families, there are more boys than girls, but in the two-child families, there are more girls than boys (some girl-girl, some girl-boy, no boy-boy). For expected gender *counts*, all kids get weighed the same, and the two skews cancel each other out. But for the expected gender *ratio*, all *family* outcomes get weighed the same, which means the single-kid families get twice as much “weight per child”, which skews the ratio in favor of boys.
40.5% may be right, but your table doesn’t show it; it may be there implicitly somehow, but it isn’t shown. The table shows 24 Hs that are followed by something. 12 of those somethings are Hs, and 12 are Ts. Can you show the calculation that produces 40.5%?
Brian/15: Right. I shouldn’t have said *first* head. I should have said *random* head. Then it works.
Robert Simmons:
Can you show the calculation that produces 40.5%?
40.5% is the average of the percentages in the final column.
I don’t know when I first read about the hot hand fallacy. Probably was something like 15 or 20 years ago in one of those pop-science books.
I remember thinking that it would be completely crazy if this result were correct. How could million dollar coaches commit such a simple error? I thought basketball coaches had to know more about this issue than some scientist in the lab, no matter how brilliant he/she was.
In a sense, knowing that the hot-hand fallacy is itself a fallacy is reassuring. It means that markets (in this case, labor markets) work better than we thought.
PS Thanks for making this post and for forcing me to read the posts about the boys-girl ratio. It took me at least an hour to fully understand the point, but it was really cool when I finally got it.
Bennett (#17)
The part I can’t get through my head is why the expected ratio must weigh all families equally. Why would we take averages of averages?
I don’t know what “expected ratio” means here and why it’s ever different from the number of girls/number of people. I have waded through all the relevant posts but I haven’t seen a dumbed down definition of what expected ratio actually means. So many of Steve’s interesting puzzles boil down to different interpretations of phrases like this.
For what its worth here is how I finally got my head around the “hot hands” bit.
I imagined a 16-sided dice each side of which contains one of the combinations of “hits” and “misses” from a series of 4 throws.
If you added up all the “HH” and “HM” from a long series of throws you would end up with the 40% ratio of HH to HH +HM shown in the post (if you added up all the “H” v totals throws you would still get 50%).
To make it even simpler – if instead of containing the combinations if H”H and “M”s the dice just contained the % of “HH” for each combination (100,67,50,50,50…etc), then its even easier to see that you would average 40% “HH” in the long run if the throws are random.
If a dice thrower was able to consistently hit 50% “HH” as a % of “HH” + “HM” then the dice must be biased or he/she has a technique to throw the sides with a higher % more frequently than would happen with random throws.
One way to intuitively get it is that the equally likely “HHH” and “THH” contribute the same amount of 100% to the average fractional value, even though HHH represents an extra shot made. Thus, we would expect a sub .5 fraction of HH relative to HT
Pat #22:
The expected ratio in my example is not weighing all families equally in a population of multiple families.
It’s taking a hypothetical population of one family (capped at two kids max), and taking all the possible outcomes, and weighing each of those outcomes in proportion to the probability of that outcome. (But outcomes with 2 kids do not get any disproportionate weight due to having 2 kids.)
That’s how you compute the “expected ratio” in a population of one family.
(In a population with multiple families — which I think is what you’re asking about — you are correct that you would not weigh families equally when computing the expected ratio; you’d just calculate the expected ratio of all boys to all girls. My one-family example was just a simple way to show that you can have E(boys) = E(girls) AND still have E(ratio) be something other than 50%.)
Perhaps the whole hot hands paradox can be simply summed up as follows:
1) If you have multiple sets of numbers S1, S2, etc., and if you take the average of each set and then take the average of those averages, you may get something different from if you take the average of all elements individually. For example if S1 = {0} and S2 = {1,1}, then the average of averages is avg(0,1) = 0.5, but the average of elements is avg(0,1,1) = 0.66. The setwise average is more biased toward the values in the smaller sets, and the elementwise average is more biased toward the values in the larger sets.
2) If looking at equal-length H/T sequences, and the sets you care about are the Hx pairs, then the more HT pairs the sequence contains, the *smaller the set is* (because it has fewer heads and thus fewer opportunities to form an Hx sequence).
3) Therefore, if you ask “What proportion of Hx sequences are HH”, the elementwise average is 0.5, but the setwise average will be biased in favor of the smaller sets, which contain more HT sequences, so the setwise average proportion of HH sequences will be something smaller than 0.5.
Pat: For *any* random variable, the expected value is defined to be the average of all possible values, weighted by their probabilities. So for example, if you throw a fair die, the expected value is the average of 1,2,3,4,5, and 6, all weighted equally. That is, it’s 3.5.
When you throw a die, the expected outcome is always 3.5, though the actual outcome could be 1,2,3,4,5 or 6.
http://www.thebigquestions.com/2010/12/22/a-big-answer-2/
Post number 100 at the link above really helped me get the gist of this problem mentally, even if I couldn’t really technically do the math on paper. Thank you Harold.
Referring to the original problem from five years ago, I think what helps me is to imagine a country with, say, 100 couples.
Then I imagine that there is some non-zero probability that the mix of the first batch of children is, say, 80 boys and 20 girls. I then realize that the same probability applies to an alternative state of the world for the first batch being 20 boys and 80 girls. It’s symmetrical for the initial batch. But for the second batch of children, if you have 80 couples that had a boy with the first batch, then that’s 80 couples that have contributed a lot of boys to the ratio that are now lost to contribute anything else. So it is hard for the remaining 20 couples to catch the ratio “back up” so to speak, even IF they have all girls in their second batch, which is highly unlikely. On the other hand, when you look at the mirror image initial batch/scenario of 80 girls and 20 boys, your future states from here are not the mirror image since you are allowing the 80 people who had a girl to keep trying. Since you’re allowed to keep trying based on sex of the child, when you consider all of the possible states of the world, it makes sense that it’s not a 50/50 expectation. It’s this asymmetry that I think is at the heart of this problem.
Steve #27
I understand your dice example but I don’t understand why we would do averages of averages ignoring family size. Your dice example doesn’t create different size groups of dice and average the averages of each group. I know what an expected value is but is that the same thing as expected ratio? If so, the ER for dice is 3.5 and the ER for coin with 0 on one side and 1 on the other is 0.5.
I’m not trying to be difficult because I’m sure I’m missing something.
Thanks for this post! I became aware of the debunking of the debunking of the hot hand when Tyler Cowen linked to it a few months ago. I actually popped open an Excel workbook and worked out the 40.5% result. I even thought, “Hmm, this is vaguely similar to that Google puzzle Landsburg posted a few years ago” and thought no more about it. When I read the title of your post, I knew what you were going to say. Good stuff.
Pat:
Each country where people follow the “keep having kids till you have a boy” rule is one throw of the dice. Each throw of the dice produces one number (the ratio of girls to (girls+boys) in that country.
We want to know the ratio of girls to girls+boys in the average country, just as we want to know the outcome of an average dice roll. Expected value is a another word for average.
(You might say, well, no, I want to know the ratio in a *particular* country, not the average country. But that’s of course hopeless, just as it’s hopeless to predict the outcome of a particular dice roll. We *can* predict averages; we can’t predict individual outcomes.)
I’m sure it must be frustrating to keep explaining this to people like me who aren’t getting it so I appreciate your persistence and patience. Thank you!
What I find fascinating is that Steven in another post said that he’s given the Monty hall problem to some of the best mathematicians in the world and some have struggled for days to get it. To me Monty Hall is easier to visualize than this problem, at least in the sense that it’s possible that the expected ratio could be something other than 50%. Anytime someone is confused about Monty Hall, I simply tell them to imagine instead of 3 shells, make it 100, and the dealer opens all but the winning door. You’re bound to get it then that you’re always better off switching your selection.
Pat, if I’m understanding your questions correctly I think maybe the source of the confusion is a comment by Bennett Haselton (17) where he says:
“But for the expected gender *ratio*, all *family* outcomes get weighed the same, which means the single-kid families get twice as much “weight per child”, which skews the ratio in favor of boys.”
To which you responded (22):
“The part I can’t get through my head is why the expected ratio must weigh all families equally. Why would we take averages of averages?”
Bennett replies in (25) explaining that he wasn’t saying in multi-family countries you would weight each family equally, he was talking about a single family with various possible outcomes.
If I’m reading your subsequent question about “averages of averages” and “ignoring family size” correctly, this is still stemming from a misunderstanding of Bennett’s original post.
In the original problem there is no equal weighting of families — the problem simply asks for the (expected) ratio of girls in the (entire) population. As math_geek says in (14), this is E(G/(G+B)) where G and B are the total number of girls and boys for the country.
As a brief example, suppose there is a 2-family country and in this particular case the resulting families turn out to be:
1)B
2)GGGGB
Then the ratio we’re interested in, G/(G+B) is 2/3 (in this specific result of one roll of the dice, so to speak). The ratio is not an average of the individual families ratios.
I understand the GB problem, and I now see how you got the 40.5% answer here. What I’m struggling with is why anyone should care about the 40.5% answer. Yes, in 40.5% of the sequences there’s an H-streak. So what? It’s still the case that all H’s are followed by another H 50% of the time.
I might make a bet on something like this, but if I’m a coach or player it’s really not relevant to anything (at least that I can see). Could you describe a scenario where this knowledge would be useful to in-game decision-making, or picking players, or something like that?
Robert Simmons (36)
“in 40.5% of the sequences there’s an H-streak. So what? It’s still the case that all H’s are followed by another H 50% of the time”
The point is that now we know basketball players don’t behave like coins, since they had H-streaks of around 50% which GVT mistakenly identified as coin-like behavior. So for them, H follows H more than 50% of the time.
Here is my simplified attempt to understand the b/g thing.
Imagine a planet with a very high number of single family countries who can have up to 3 kids and stop at the first boy.
You could construct an 8-sdied dice that accurately reflected the distribution of girls/boys (50% b only, 25%b/g, 12.5% for both ggb and ggg
The sisde would be as follows. The number in brackets is the proportion of girls)
b(0)
b(0)
b(0)
b(0)
gb(0.5)
gb(0.5)
ggb(0.67)
ggg(1)
If you counted the number of b and g’s on the dice you get 7 of each to reflect the 50% chance of either sex.
But if rolled the dice lots of times you would get an average female ratio of 33% not 50%.
If you introduce multiple family countries then the dice you built would still always contain an equal # of b’s and g’s, and the average ratio of girls for long sequences of throws (if the aveage number of families in a country is high enough) would increase until it approached (but never quite reached) 50%.
If you remove the 3-kids rule you can no longer build a dice but the logic remains the same.
@Robert(36): You are right, there is no reason anyone should care about the 40% answer, just as there is no reason to care about such answers to the girl-boy problem.
The interesting thing here is that some very famous research relied on that 40% answer, and nobody noticed for 30 years that it was an error.
So you’re saying the 40.5% answer is relevant because they were looking at the problem in a wrong way? Ok, but I say let’s stick with the right one from now on. If H is followed by H >50% of the time, there may be a real hot hand phenomenon. Let’s also look at what follows HH, and HHH, and so on.
For testing the hot hand hypothesis, you could do it a lot of ways. The null hypothesis is that each shot follows some shooter’s percentage, and is independent of previous shots. You define some measure of success, and see if real-world data does significantly better than the null hypothesis.
You can use some screwy weighting if you want, but you have to do the same with the null hypothesis, and compare. Apparently no one noticed that the null hypothesis might only give 40.5% for the particular screwy test being used.
I think I finally understand why the answer to the Google question is not 50%. It is very unintiuitive, but thanks to the simplified example at http://www.thebigquestions.com/alt.txt it clicked.
But now I’m wondering when it ever makes sense to talk about an expected value of a fraction. It seems like it’s just a meaningless number (meaningless since most humans will not understand it’s real meaning).
So my question is: what is a good example where you want to know the expected value of a fraction?
Mirolyub Hristov:
So my question is: what is a good example where you want to know the expected value of a fraction?
If you want to correctly interpret the “hot hand” statistics (i.e. if you care about whether hot hands exist), then you’ll want to know the expected value of the fraction #HH/(#HH+#HT) .
Well, now I feel silly. When I finally understood the answer to the Google question I decided that the expected value of fraction must be useless except in tricky puzzles. Sorry for not reading your post properly before posting. This is a pretty good example.
Correct me if I’m wrong but the fraction of girls depends on the size of the country, the larger the country the closer the faction gets to 50%. Surely if the country is infinitely large and people can have an infinite number of children the fraction of girls would be 49.9 recurring. Since 0.9 recurring equals 1 the fraction is 50%.
Richard R:
Correct me if I’m wrong
You’re wrong.
If the population is infinite, then the ratio you’re attempting to form has an infinite denominator and so is undefined.
Re: 45 and 46, Isn’t the general point valid though? Can’t you make E(G/(G+B)) arbitrarily close to (but less than) 50% by making the number of families arbitrarily large?
Brian: Richard R asked about the ratio of the limiting values of G and G+B, which is undefined. You are asking about the limiting value of the ratio, which is of course a very different thing and is indeed 1/2.
A fallacy tangentially related to this one:
On the first day of his justice class at Harvard, Michael Sandel would poll the students as to how many were the oldest in their families. He always found that the fraction of “oldests” was larger than the general population, and concluded that the accident of birth order gave them an edge over younger siblings in getting into Harvard. What was his mistake? (Answer at http://personal.lse.ac.uk/calel/Millner%20and%20Calel%20(2010).pdf )
Jonathan Weinstein: Nice find! Thank you.
The Millner and Calel paper is interesting. Wjilst I am sure they have got it correct about birth order effect, I am not sure they have got Sandel’s proposal correct.
“Sandel seems to be inferring that the fact that so many Harvard under-graduates are 1st born suggests that birth order has a strong effect on academic effort.”
Since they say he “seems to be inferring” I can conclude that Sandel does not actually make such a claim explicitly.
However, Sandel is actually claiming that “Rawls suggests that all three of these theories base just distributive shares on morally arbitrary chance endowments { whether of birth, property,or genetically inherited natural abilities).”
Being first born of many or an only child is just such a factor, so whether it is a birth order or a low fertility effect, it is still a morally arbitrary endowment.
“… a new paper by Joshua Miller and Adam Sanjurjo (call them M and S)….”
Can someone here explain something about Table 1, PDF page 5, of the above referenced MS paper (the subject of this post).
The bottom row, Expected Value (fair coin), shows .40 as the arithmetic average of both A) p(H|H) and B) E[p(H|H) | nH]. For the first (A), the .40 appears to be the result of taking 1/14th of 5 & 2/3rds (.4048 rounded to .40). For the second (B), .40 appears to be the result of taking 1/5th of 2 (.40).
The 1/14th used in the first (A) disregards the top subset of runs (TTTT) and the first run (TTTH) of the nest subset (1 head showing – TTTH). If one or both runs are not disregarded, then the numerator (5 & 2/3rds (A))would be divided by either 15 or 16 (not 14). If so, they round to .38 or .35 respectively.
However, the 1/5th used in B does regard the top subset (TTTT). If it were disregarded, as it is in the first (A), and only the 4 subsets by which the B numerator (2) is calculated were used, the arithmetic average of E[p(H|H) | nH would be .50.
Why is the top subset (TTTT) disregarded in A but not in B?
Most interestingly, Sandel’s finding could even be consistent with a *last-born* advantage.
That is a very cogent explanation of a flaw in the approach used in the basketball paper (which I have not yet read) but…
As you no doubt know the NY Times had a piece on this topic in their Sunday edition.
Picking up on the theme set by your response to John Faben (1:02 PM Oct 7), how would you assess this statement by the Times summing up the new paper?
“In a study that appeared this summer, Joshua B. Miller and Adam Sanjurjo suggest why the gambler’s fallacy remains so deeply ingrained. Take a fair coin — one as likely to land on heads as tails — and flip it four times. How often was heads followed by another head? In the sequence HHHT, for example, that happened two out of three times — a score of about 67 percent. For HHTH or HHTT, the score is 50 percent.
Altogether there are 16 different ways the coins can fall. I know it sounds crazy but when you average the scores together the answer is not 50-50, as most people would expect, but about 40-60 in favor of tails.”
Well, yes, it does sound crazy. Heads was followed by heads half the time, as expected. However, the statement about the (unweighted) averages is also true. Weighting each of the sixteen flip sequences by the number of trials it produces (a ‘trial’ being an H in any of the first three positions) produces the expected 50/50 result.
If people intuitively expect an unweighted average to be meaningful, well, that is why mathematicians (and bond traders) earn the big bucks.
But in the chart you depict, HHHH has a 100% success rate and goes in to the calculation with a weight of 1 (as per the original authors). The equally likely TTHH also has a 100% success rate and a weight of 1.
But HHHH walked a tightrope, since any of the first three heads could have been followed by a failure. TTHH had only one chance to do or die. So the “intuitive” idea that we ought to weight each of the sixteen flip sequences by the number of trials they produced (and hence, the opportunities for success or failure) is hardly unreasonable.
And yes, reweighting does lead to a 50/50 result.
The Times continues (my emphasis):
“In an interesting twist, Dr. Miller and Dr. Sanjurjo propose that research claiming to debunk the hot hand in basketball is flawed by the same kind of misperception. Studies by the psychologist Thomas Gilovich and others conclude that basketball is no streakier than a coin toss. For a 50 percent shooter, for example, the odds of making a basket are supposed to be no better after a hit — still 50-50. But in a purely random situation, ***according to the new analysis, a hit would be expected to be followed by another hit less than half the time***. Finding 50 percent would actually be evidence in favor of the hot hand. If so, the next step would be to establish the physiological or psychological reasons that make players different from tossed coins.”
That seems to mis-state the supportable conclusion.
Not to be a grind, but I have the same question about the lead paragraph of the working paper in question (my emphasis):
“Jack takes a coin from his pocket and decides that he will flip it 4 times in a row, writing down the outcome of each flip on a scrap of paper. **After he is done flipping, he will look at the flips that immediately followed an outcome of heads, and compute the relative frequency of heads on those flips.*** Because the coin is fair, Jack of course expects this empirical probability of heads to be equal to the true probability of flipping a heads: 0.5. ***Shockingly, Jack is wrong.*** If he were to sample one million fair coins and flip each coin 4 times, observing the conditional relative frequency for each coin, on average the relative frequency would be approximately 0.4”
I am a bigger fan of Jack then they are. Jack has the right answer to the question he asked himself, since we all agree that even in the table shown, HH and HT are equally likely.
The authors then reframe the question to one Jack never asked, adopt a weighting scheme that might very well have not seemed reasonable to him, and conclude that Jack’s intuition is wrong. Hmmm… Not to be judgmental but I don’t think they know Jack.
All that said, if the basketball people really did simply put different players in a small sample trial and then calculate the unweighted results as described, then this new rebuttal appears to be spot on.
One last question (said Columbo…)
In the linked Gilovich article the closest I can find to the data being partitioned into blocks of four is this (with the 76ers from page 7, repeated with Cornell students on p 12):
START
“To obtain a more sensitive test of stationarity, or a constant hit rate, we partitioned the entire record of each player into nonoverlapping sets of four consecutive shots. We then counted the number of sets in which the player’s performance was high (three or four hits), moderate (two hits), or low (zero or one hit). If a player is occasionally hot, then his record must include more high-performance sets than expected by chance.
The number of high, moderate, and low sets for each of the nine players were compared to the values expected by chance, assuming independent shots with a constant hit rate (derived from column 5 of Table 1). For example, the expected proportions of high-, moderate-, and low-performance sets for a player with a hit rate of 0.5 are 5/16, 6/16, and 5/16, respectively. The results provided no evidence for nonstationarity, or streak shooting, as none of the nine x2 values approached statistical significance.
This analysis was repeated four times, starting the partition into consecutive quadruples at the first, second, third, and fourth shot of each player’s shooting record. All of these analyses failed to support the nonstationarity hypothesis.”
END
That is obviously the 1-4-6-4-1 binomial distribution with the two tails combined. Presumably a streaky shooter would show a skewed distribution. (Later I explain how to boil water…)
As I understand Gilovich (and using the Heads/Tails notation), HHHT, HHTH, HTHH and THHH would all score equally as a high success rate. Yet in the refuting paper, those four results produce different scores, which is the basis for the refutation. Que pasa?
HHHT: 67%
HHTH: 50%
HTHH: 50%
THHH: 100%
Josh, #34,
“Anytime someone is confused about Monty Hall, I simply tell them to imagine instead of 3 shells, make it 100, and the dealer opens all but the winning door. You’re bound to get it then that you’re always better off switching your selection.”
That would not have worked for me. When I did not get the Monty Hall problem, I had assigned an informational value of zero to opening of doors. I had not connected the door-selections and the door-openings. In other words, I thought about selecting a door to open as the same whether I selected before or after the doors were opened. That was incorrect, but that is how I thought about it. If I assigned 0 informational value to opening 1 door, I would have assigned 0 informational value to opening 99 doors also.
I think there’s a mistake in footnote 1 from the paper. I don’t see how the expected payout of the game is $-0.71.
The expected relative frequency of HH/(HH+HT) is 17/42 = 40.47619%, which Steve rounds up to 40.5% in his chart but the authors round down to 40% in their chart. But if you’re only interested in whether HH is strictly greater than HT or HH is strictly less than HT, you simply count the sequences for which HH/(HH+HT) is neither 50% nor undefined. There are four sequences where HH is strictly greater than HT (TTHH, THHH, HHHT, and HHHH) and six where HH is strictly less than HT (TTHT, THTT, HTTT, THTH, HTTH, and HTHT). So, when invalid sequences result in a redo as per the rules, the chance that HH is strictly greater than HT is 40% (exactly, not approximately), and the expected payout of the game is (10*4/10 + 0*6/10) – 5 = -$1.
If the game paid out when HH>=HT on the other hand, the four possible 50-50 outcomes would count as a win instead of a redo, and the expected payout of the game would be (10*8/14 + 0*6/14) – 5 = $0.7142857, which is the only way I get something that looks like their answer, but it has the wrong sign.
@ Kyle #58
Try it with “the four possible 50-50 outcomes” each counting has a half win for both sides. Now you have (10*6/14 + 0*8/14) – 5 = -$0.7142857.
But I read their footnote as saying a 50-50 outcome is no play, no game:
“…[I]f the relative frequency is exactly equal to 0.5, or if no flip is immediately preceded by a heads, then a new sequence of 4 flips is generated.”
Consider the scenario with coin flips instead of births. Let’s say I’m making rows of coins. For each row, I flip a coin and set it down. If it’s heads, I move on to the next row. If it’s tails, I continue flipping and setting down the coins until I get a heads.
My assumption is these two questions are different:
a) For any given number of coins on the table, what percentage will likely be tails?
b) Of the coins on the table at any given time, what percentage will likely be tails?
I believe the answer to B is 50%, and I suspect that whoever wrote the question attributed to Google intended to write a parallel scenario using births. However, I think it’s quite reasonable to interpret “fraction of the population” as meaning scenario A, in which case Prof. Landsburg’s calculation would be the appropriate one to use.
I know you don’t want to “re-litigate” but I was just reviewing the link someone posted above to the simplified example, here: http://www.thebigquestions.com/alt.txt
As Ellenberg mentioned, you’ve got a divide by zero error there. The weighted average of probabilities as you’ve defined it is actually (1/4 * 0/2) + (1/8 * 1/3) etc.
Is it just the table that’s incorrectly illustrating the more complex mathematical formula or is there something else going on?
Sorry to keep asking questions but I’m confused. Can you explain again how you got the 40.5%?
It appears that you’re saying (3 / (3 + 0)) + (2 / (2 + 1)) + … + ( 0 / (0 + 2)) + (0 / (0 + 1)) + (0 / (0 + 1)) + (0 / (0 + 0)) + (0 / 0 + 0))) / 14 = 40.5
Since no one else has challenged this I assume there’s some mathematical rationale that allows you to do this, although it appears to be a violation of a fundamental rule of arithmetic. I’d always thought that “0%” was an advertising ploy, not an actual number – that the figure in line 6, above, should be plain old 0, not 0/100.
I’m not sure that the M&S result does in fact support your analysis of the Boys & Girls problem.
If the calculation shows that the expected probability of HH is 40.5%, then I think that suggests we’re asking the wrong question in the first place. If our hypothesis is that each throw has an independent 50% chance of success, then the answer to the right question should be 50%, and the empirical observation should show a success rate > 50%, because, in fact, our hypothesis is incorrect (based on M&S) and the chance of success is > 50%.
Same thing with Jack the coin flipper – the conditional probability of the question he’s asking is 40% because he isn’t asking the question he thinks he’s asking.
So too with the boys & girls problem – if we think of it in terms of “streaks,” I believe you’re saying that while we might expect the length of the average streak to be 2 (each single M gets balanced by a corresponding GGM), in fact the average streak length is less than 2. That’s certainly true – but I suggest it’s the right answer to the wrong question, because “fraction of population” isn’t calculated by averaging streak length but is simply E(Female population of country) / E(total population of country).
Looks like Jordan Ellenberg has gotten in on the action:
http://www.slate.com/articles/health_and_science/science/2015/11/solution_to_coin_flip_paradox_when_to_bet_heads_or_tails.html